This is a very long post, but it also covers what are probably the classes (well, array_view at least) that you will use the most with C++ AMP, so I hope you enjoy it!
Overview
The concurrency::array and concurrency::array_view template classes represent multi-dimensional data of type T, of N dimensions, specified at compile time (and you can later access the number of dimensions via the rank property). If N is not specified, it is assumed that it is 1 (i.e. single-dimensional case). They are rectangular (not jagged).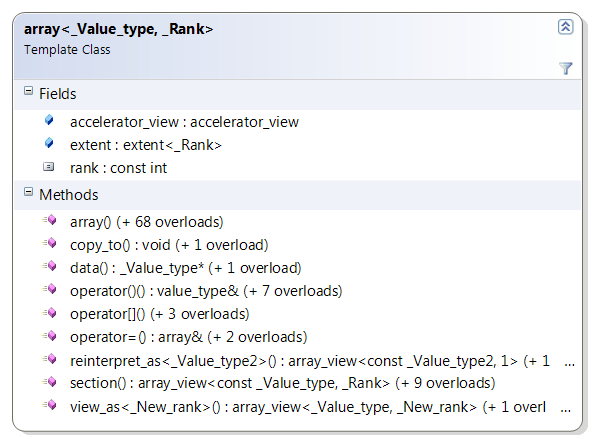
The difference between them is that array is a container of data, whereas array_view is a wrapper of a container of data. So in that respect, array behaves like an STL container, whereas the closest thing an array_view behaves like is an STL iterator (albeit with random access and allowing you to view more than one element at a time!).
The data in the array (whether provided at creation time or added later) resides on an accelerator (which is specified at creation time either explicitly by the developer, or set to the default accelerator at creation time by the runtime) and is laid out contiguously in memory. The data provided to the array_view is not stored by/in the array_view, because the array_view is simply a view over the real source (which can reside on the CPU or other accelerator). The underlying data is copied on demand to wherever the array_view is accessed. Elements which differ by one in the least significant dimension of the array_view are adjacent in memory.
array objects must be captured by reference into the lambda you pass to the parallel_for_each call, whereas array_view objects must be captured by value (into the lambda you pass to the parallel_for_each call). After you are done reading this post, feel free to visit another post dedicated to capturing data.
Creating array and array_view objects and relevant properties
You can create array_view objects from other array_view objects of the same rank and element type (shallow copy, also possible via assignment operator) so they point to the same underlying data, and you can also create array_view objects over array objects of the same rank and element type e.g.
array_view<int,3> a(b); // b can be another array or array_view of ints with rank=3
Note: Unlike the constructors above which can be called anywhere, the ones in the rest of this section can only be called from CPU code.
You can create array objects from other array objects of the same rank and element type (copy and move constructors) and from other array_view objects, e.g.
array<float,2> a(b); // b can be another array or array_view of floats with rank=2
To create an array from scratch, you need to at least specify an extent object, e.g. array<int,3> a(myExtent);
. Note that instead of an explicit extent object, there are convenience overloads when N<=3 so you can specify 1-, 2-, 3- integers (dependent on the array's rank) and thus have the extent created for you under the covers. At any point, you can access the array's extent thought the extent property. The exact same thing applies to array_view (extent as constructor parameters, incl. convenience overloads, and property).
While passing only an extent object to create an array is enough (it means that the array will be written to later), it is not enough for the array_view case which must always wrap over some other container (on which it relies for storage space and actual content). So in addition to the extent object (that describes the shape you'd like to be viewing/accessing that data through), to create an array_view from another container (e.g. std::vector) you must pass in the container itself (which must expose .data() and a .size() methods, e.g. like std::array does), e.g.
array_view<int,2> aaa(myExtent, myContainerOfInts);
Similarly, you can create an array_view from a raw pointer of data plus an extent object.
Back to the array case, to optionally initialize the array with data, you can pass an iterator pointing to the start (and optionally one pointing to the end of the source container) e.g.
array<double,1> a(5, myVector.begin(), myVector.end());
We saw that arrays are bound to an accelerator at creation time, so in case you don’t want the C++ AMP runtime to assign the array to the default accelerator, all array constructors have overloads that let you pass an accelerator_view object, which you can later access via the accelerator_view property.
Note that at the point of initializing an array with data, a synchronous copy of the data takes place to the accelerator, and then to copy any data back we'll see that an explicit copy call is required. This does not happen with the array_view where copying is on demand...
refresh and synchronize on array_view
Note that in the previous section on constructors, unlike the array case, there was no overload that accepted an accelerator_view for array_view. That is because the array_view is simply a wrapper, so the allocation of the data has already taken place before you created the array_view. When you capture an array_view variable in your call to parallel_for_each, the copy of data between the non-CPU accelerator and the CPU takes place on demand (i.e. it is implicit, versus the explicit copy that has to happen with the array). There are some subtleties to the on-demand-copying that we cover next.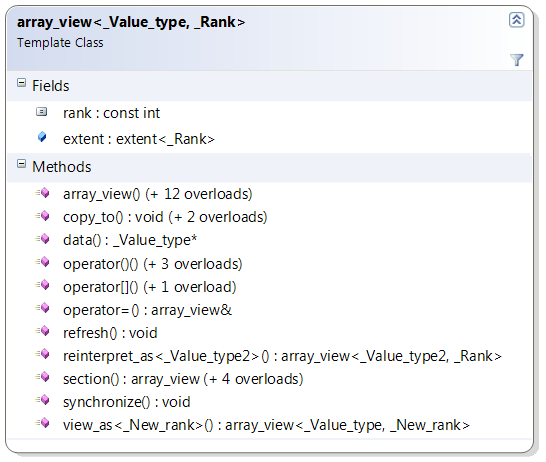
The assumption when using an array_view is that you will continue to access the data through the array_view, and not through the original underlying source, e.g. the pointer to the data that you passed to the array_view's constructor. So if you modify the data through the array_view on the GPU, the original pointer on the CPU will not "know" that, unless one of two things happen:
- you access the data through the array_view on the CPU side, i.e. using indexing that we cover below
- you explicitly call the array_view's synchronize method on the CPU (this also gets called in the array_view's destructor for you)
Conversely, if you make a change to the underlying data through the original source (e.g. the pointer), the array_view will not "know" about those changes, unless you call its refresh method.
Finally, note that if you create an array_view of const T, then the data is copied to the accelerator on demand, but it does not get copied back, e.g.
array_view<const double, 5> myArrView(…); // myArrView will not get copied back from GPU
There is also a similar mechanism to achieve the reverse, i.e. not to copy the data of an array_view to the GPU.
copy_to, data, and global copy/copy_async functions
Both array and array_view expose two copy_to overloads that allow copying them to another array, or to another array_view, and these operations can also be achieved with assignment (via the = operator overloads).
Also both array and array_view expose a data method, to get a raw pointer to the underlying data of the array or array_view, e.g. float* f = myArr.data();
. Note that for array_view, this only works when the rank is equal to 1, due to the data only being contiguous in one dimension as covered in the overview section.
Finally, there are a bunch of global concurrency::copy functions returning void (and corresponding concurrency::copy_async functions returning a future) that allow copying between arrays and array_views and iterators etc.
Note that for array, all copying described throughout this post is deep copying, as per other STL container expectations. You can never have two arrays point to the same data.
indexing into array and array_view plus projection
Reading or writing data elements of an array is only legal when the code executes on the same accelerator as where the array was bound to. In the array_view case, you can read/write on any accelerator, not just the one where the original data resides, and the data gets copied for you on demand. In both cases, the way you read and write individual elements is via indexing as described next.
To access (or set the value of) an element, you can index into it by passing it an index object via the subscript operator. Furthermore, if the rank is 3 or less, you can use the function ( ) operator to pass integer values instead of having to use an index object. e.g.
array<float,2> arr(someExtent, someIterator); //or array_view<float,2> arr(someExtent, someContainer);
index<2> idx(5,4);
float f1 = arr[idx];
float f2 = arr(5,4); //f2 ==f1
//and the reverse for assigning, e.g.
arr(idx[0], 7) = 6.9;
Note that for both array and array_view, regardless of rank, you can also pass a single integer to the subscript operator which results in a projection of the data, and (for both array and array_view) you get back an array_view of rank N-1 (or if the rank was 1, you get back just the element at that location).
Not Covered
In this already very long post, I am not going to cover three very cool methods (and related overloads) that both array and array_view expose: view_as, section, reinterpret_as. We'll revisit those at some point in the future, probably on the team blog.